Spiking neural network (SNN) utilizes spike trains for information processing among neurons, which is more biologically plausible and widely regarded as the third-generation artificial neural network (ANN). It has the potential for effectively processing spatial-temporal information and has the characteristics of lower power consumption and smaller calculation load compared with conventional ANNs. In this work, we demonstrate the feasibility of applying SNN to classify tactile signals collected by a bionic artificial fingertip that touches a group of real-world metal surfaces with different roughness levels. A two-layer SNN is adopted and trained using an unsupervised learning method with spike-timing-dependent plasticity (STDP). Experiments show that the trained SNN can categorize the input tactile signals into different surface roughness of metal textures with more than 80% accuracy. This work lays the foundation of applying SNNs to more complex tactile signal processing in robotics, manufacturing, and other engineering fields.)
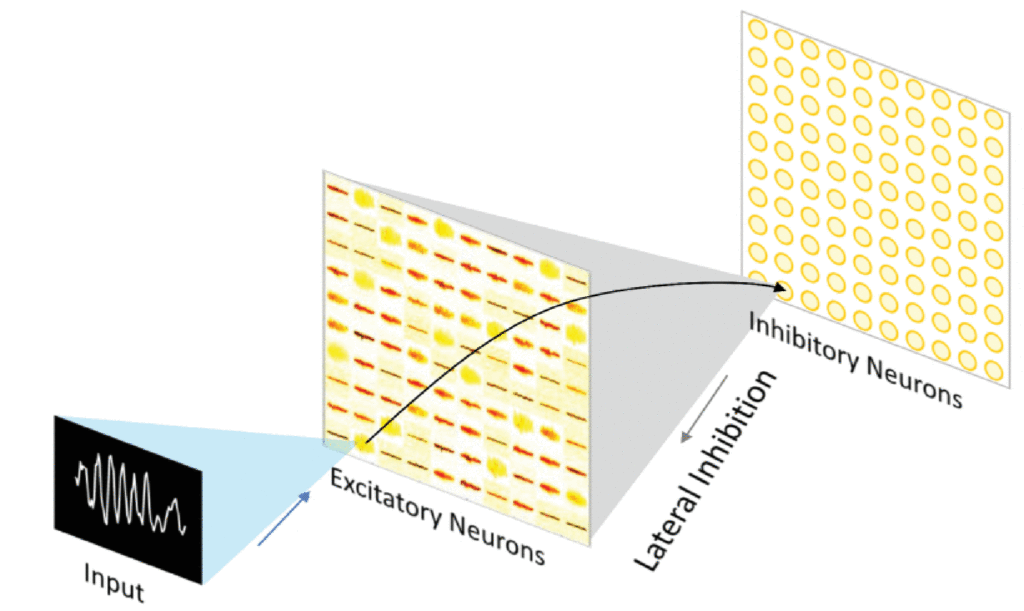
In this paper, we explore the possibility of performing tactile surface roughness discrimination with the biologically inspired SNN model. The Ra value range of sampled surfaces is from 0.4 μm to 50 μm . Ultimately, the test result shows this method can reach the highest 83.3% accuracy by the PVDF1 dataset and 79.3% by both PVDF datasets. This method is robust and suitable for real-time surface roughness discrimination. The insights gained from this study may be of assistance to developing advanced neurorobotics combined with SNN. Further work needs to be done to explore whether SNN can better identify the signal in the frequency domain and by a new encoding method.
Associate Professor Yilei Zhang is involved with this work. To read the full paper please visit the IEEE Sensors Journal, Volume 22, Issue 1